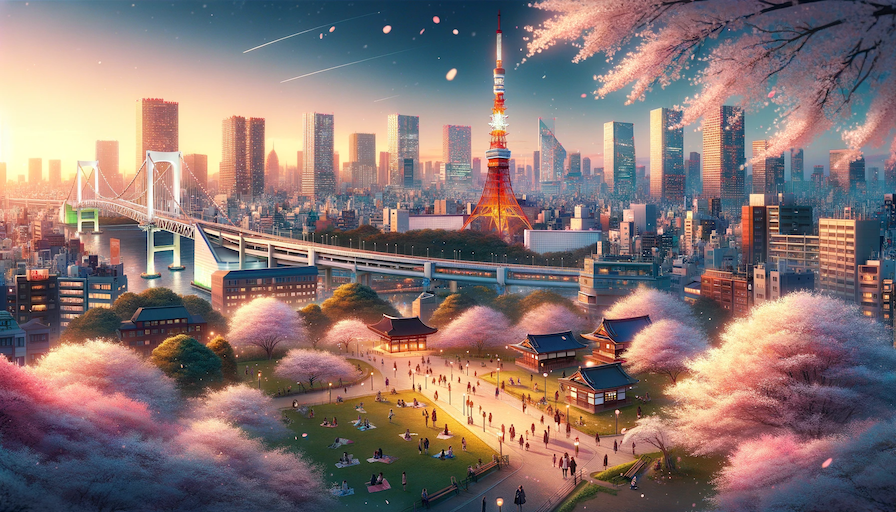
Sixth Conference on
Discrete Optimization and Machine Learning
Summer 2024 x Tokyo, Japan
Coordinates
Dates: 8th - 10th July, 2024
Place: National Graduate Institute for Policy Studies (GRIPS), Tokyo, Japan
Organizers: Antoine Deza, Sebastian Pokutta, Takashi Tsuchiya
Supported by the Center of Data Science @ GRIPS
Conference Dinner: July 8 at 6 pm at 魚真 乃木坂店 (Uoshin Nogizaka)
9 Chome-6-32 Akasaka, Minato City, Tokyo 107-0052 [map]
Accomodation
There are many hotels in the Roppongi area, e.g.,
Act Hotel Roppongi, Remm Roppongi, and Confort Inn Tokyo Roppongi.
Participants
- Salvador Abreu
- David Avis
- Jean-François Baffier
- Steffen Borgwardt
- David Bremner
- Daniel Dadush
- Antoine Deza
- Arnaud Deza
- Katsuki Fujisawa
- Jun-ya Gotoh
- Akshay Gupte
- Satoru Iwata
- Hiroshi Kera
- Matthias Köppe
- Leo Liberti
- Zhongyuan Liu
- Bruno Lourenço
- Kazuhide Nakata
- Keiyu Nosaka
- Pierre-Louis Poirion
- Sebastian Pokutta
- Ting Kei Pong
- Vera Roshchina
- Jan-Dirk Schmöcker
- Akiyoshi Shioura
- Renata Sotirov
- Masashi Sugiyama
- Akiko Takeda
- Ken'ichiro Tanaka
- Takashi Tsuchiya
- Takashi Ui
- Andrea Walther
- Dadi Wang
- Akiko Yoshise
- Yuan Zhou
Program
July 8
Time | Speaker | Talk Title (for abstract click title or see below) |
---|---|---|
09:30 - | Registration/Coffee | |
10:00 - | Session Chair: Katsuki Fujisawa | |
10:00 - | Masashi Sugiyama |
Training Error and Bayes Error in Deep Learning
|
10:30 - | Leo Liberti |
Unassigned distance geometry
|
11:00 - | Bruno Lourenço |
Projecting onto hyperbolicity cones and beyond
|
11:30 - | Akiko Takeda |
Applying Random projection techniques to large-scale optimization problems
|
Lunch Break | ||
14:00 - | Session Chair: Akiko Takeda | |
14:00 - | Katsuki Fujisawa |
Mathematical Optimization Models for Smart Factory Construction and Applications to the Field
|
14:30 - | Andrea Walther |
On the solution of nonsmooth optimization problems from retail
|
15:00 - | Jean-François Baffier |
Learning Nonlinear QUBO Models in Discrete Spaces
|
Coffee Break | ||
16:00 - | Session Chair: Leo Liberti | |
16:00 - | Daniel Dadush |
A strongly polynomial algorithm for linear programs with at most two non-zero entries per row or column
|
16:30 - | Satoru Iwata |
Discrete Optimization for Atom Mapping
|
17:00 - | Arnaud Deza |
Learn2Aggregate: Supervised Generation of Chvátal-Gomory Cuts
|
Dinner and Group Photo |
July 9
Time | Speaker | Talk Title (for abstract click title or see below) |
---|---|---|
09:30 - | Coffee | |
10:00 - | Session Chair: David Bremner | |
10:00 - | David Avis |
On the holographic cone
|
10:30 - | Renata Sotirov |
Cuts and semidefinite liftings for the complex cut polytope
|
11:00 - | Jun-ya Gotoh |
EM Algorithms for Optimization Problems with Polynomial Objectives
|
11:30 - | Jan-Dirk Schmöcker |
Hyperpath-based Route Choice and Delay Insurance Fares
|
Lunch Break | ||
13:30 - | Session Chair: Daniel Dadush | |
13:30 - | Takashi Tsuchiya |
An Analysis on Relation of Political Systems and Development of World Countries based on a Markov Model
|
14:00 - | David Bremner |
Towards a practical imperative modelling language for (integer) linear programming
|
14:30 - | Steffen Borgwardt |
An Introduction to Circuit Walks
|
Coffee Break | ||
15:30 - | Session Chair: Yuan Zhou | |
15:30 - | Matthias Köppe |
The modularized SageMath library in the DOMLverse
|
16:00 - | Ting Kei Pong |
Kurdyka-Łojasiewicz exponent for a class of Hadamard-difference-parameterized models
|
16:30 - | Hiroshi Kera |
Learning to Do Computational Algebra via Transformer
|
July 10
Time | Speaker | Talk Title (for abstract click title or see below) |
---|---|---|
09:30 - | Coffee | |
10:00 - | Session Chair: Takashi Tsuchiya | |
10:00 - | Pierre-Louis Poirion |
Random sub-manifold methods for optimization on the Stiefel manifold
|
10:30 - | Vera Roshchina |
Structure of convex sets in infinite dimensional spaces
|
11:00 - | Sebastian Pokutta |
Extending the Continuum of Six-Colorings
|
11:30 - | Akiko Yoshise |
Procrustean Data Collaboration for Cross-Silo Privacy-Preserving Machine Learning
|
Lunch Break | ||
13:30 - | Session Chair: Andrea Walther | |
13:30 - | Takashi Ui |
LQG information design
|
14:00 - | Akshay Gupte |
Subadditive duality in mixed-integer conic optimization
|
14:30 - | Antoine Deza |
Worst-case optimization instances
|
Abstracts
David Avis
The holographic entropy cone (HEC) is a polyhedral cone first introduced in the study of a class of quantum entropy inequalities closely related to the celebrated Ryu-Takayanagi prescription. It admits a graph-theoretic description in terms of minimum cuts in weighted graphs, a characterization which naturally generalizes the cut function for complete graphs. Unfortunately, no complete facet or extreme-ray representation of the HEC is known. I will survey what is known about this cone and give several open questions.
Jean-François Baffier
We propose a novel tool to learn discrete, possibly nonlinear, QUBO models through combinatorial optimization. Our approach automates the learning of constraint representations, crucial for accurately modeling optimization problems. By isolating and combining patterns from data, our method ensures scalability and robustness, successfully applying learned patterns from small instances to larger ones and performing well even with limited training data. The resulting QUBO models can be solved by various optimization methods, including Quantum Annealing, enhancing the modeling of combinatorial optimization problems in complex scenarios.
Steffen Borgwardt
We provide an introduction to the studies of circuit walks and review the past decade of research on circuit diameters and augmentation. We connect the field to classical topics such as conformal sums and combinatorial diameters, discuss the efficient computation of improving circuits, and end with some open questions.
David Bremner
Previous work on compiling algorithms to linear programs has mainly focused on theoretical issues like P-completeness and extension complexity. In this talk I will report work in progress with my PhD student Shermin Khosravi on making such compilers into practical modelling tools. In this talk I will present some techniques for substantially restructuring (and shrinking) the generated sets of inequalities. By considering the inequalities as a simulation of a register machine running a particular procedural code, we are able to go beyond what is possible with traditional compiler optimizations and solver preprocessing techniques, since they both miss part of the picture.
Daniel Dadush
We give a strongly polynomial algorithm for minimum cost generalized flow, and hence for optimizing any linear program with at most two non-zero entries per row, or at most two non-zero entries per column. Primal and dual feasibility were shown by Megiddo (SICOMP ’83) and Végh (MOR ’17) respectively. Our result can be viewed as progress towards understanding whether all linear programs can be solved in strongly polynomial time, also referred to as Smale’s 9th problem. Our approach is based on the recent primal-dual interior point method (IPM) due to Allamigeon, Dadush, Loho, Natura and Végh (FOCS ‘22). The number of iterations needed by the IPM is bounded, up to a polynomial factor in the number of inequalities, by the straight line complexity of the central path. Roughly speaking, this is the minimum number of pieces of any piecewise linear curve that multiplicatively approximates the central path. As our main contribution, we show that the straight line complexity of any minimum cost generalized flow instance is polynomial in the number of arcs and vertices. By applying a reduction of Hochbaum (ORL ‘04), the same bound applies to any linear program with at most two non-zeros per column or per row. To be able to run the IPM, one requires a suitable initial point. For this purpose, we develop a novel multistage approach, where each stage can be solved in strongly polynomial time given the result of the previous stage. Beyond this, substantial work is needed to ensure that the bit complexity of each iterate remains bounded during the execution of the algorithm. For this purpose, we show that one can maintain a representation of the iterates as a low complexity convex combination of vertices. Our approach is black-box and can be applied to any log-barrier path following method.
Antoine Deza
Abstract. Worst-case constructions have helped providing a deeper understanding of how the structural properties of the input affect the computational performance of optimization algorithms. Recent examples include the construction of Allamigeon, Benchimol, Gaubert, and Michael Joswig, for which the interior point method performs an exponential number of iterations. In a similar spirit, we investigate the following question: how close can be two disjoint lattice polytopes contained in a fixed hypercube? This question stems from various contexts where the minimal distance between such polytopes appears in complexity bounds of optimization algorithms. We provide nearly matching lower and upper bounds on this distance and discuss its exact computation. Based on joint work with Shmuel Onn (Technion), Sebastian Pokutta (ZIB), and Lionel Pournin (Paris XIII).
Arnaud Deza
Recent years have witnessed a number of research efforts to enhance the strength of cutting plane subroutines, specifically cutting plane selection using machine learning (ML). This work has a similar goal by aiming to assist a computationally expensive separator, the Chvátal-Gomory (CG) cut generator, in integer programming (IP) solvers using data-driven analysis. We embed ML into the generation of valid inequalities and show how optimized cut selection can be incorporated into the learning phase for cut generation. Our ML framework consists of a binary classification model trained to identify a subset of useful constraints for the aggregation step in CG cut generation. Although we only focus on CG cuts, our approach can be extended to other families of aggregation-based cuts. Preliminary experiments on synthetic IP instances demonstrate promising results regarding the validity of ML research for cut generation.
Katsuki Fujisawa
By utilizing the latest mathematical and information technologies, efforts are underway to build digital twins (encompassing both physical and cyber spaces) to facilitate the resolution of various issues facing cities, regions, and the industrial sector. This talk will provide an explanation of research on the latest mathematical optimization models for the realization of digital twins, along with specific applications in the industry. Additionally, an introduction to a smart factory construction project currently in progress will be presented.
Jun-ya Gotoh
EM (Expectation-Maximization) algorithm is a popular algorithm often used in maximum likelihood estimation of statistical models when they include latent random variables. With the focus on its MM (Majorization-Minimization) optimization features, a new algorithmic scheme based on EM algorithm is presented for solving general (not necessarily statistical) optimization problems by introducing certain probability distributions. We show that by choosing a suitable distribution from the exponential family, optimization problems with polynomial objective functions, which include linear program (LP) and quadratic program (QP), can be embedded in the EM scheme. Specifically, with a normal distribution, the EM algorithm for the unconstrained minimization of a convex quadratic objective function turns out to be a natural gradient descent without line search. For minimizing a polynomial objective function over a rectangle or a simplex, the EM algorithm with a binomial or multinomial distribution, respectively, is shown to become an interior point algorithm which is reduced to a natural gradient descent without line search. As further extensions to the case where a natural gradient descent without line search is no longer available, new interior point algorithms are presented for polynomial optimization over a polytope, LP, and QP, based on EM and generalized EM algorithms. The presented algorithms for the extensions are somewhat conceptual in that their sub-procedures require solving a nonlinear convex optimization, and some practical strategies are discussed. (This is a joint work with Kensuke Asai.)
Akshay Gupte
We will talk about recent progress in obtaining exact duals for mixed-integer conic programs. Contrary to other studies, our duals circumvent the use of directional derivatives for continuous variables. This allows us to create an extended formulation of the closure convex hull. We will also use duality for checking feasibility of the mixed-integer program. This work is part of a collaboration grant with Andrew Schaefer at Rice University.
Satoru Iwata
Given a pair of molecular structures of reactants and products, determine a natural one-to-one correspondence of the atoms. This problem has been investigated under the name of atom mapping. The atom mapping itself is not the final target task. It is used for assisting chemists in design and discovery of chemical reactions. For this purpose, it is not necessarily desired to determine one “optimal” solution. Instead, it is more desirable to suggest a reasonable number of possible solutions with guarantees. In this talk, we discuss how to model the atom mapping in terms of discrete optimization and how to develop a practically efficient algorithm that provides solutions with theoretical guarantees. This is joint work with Keisuke Katayama at the University of Tokyo together with Seiji Akiyama and Yuuya Nagata at ICReDD, Hokkaido University.
Hiroshi Kera
Computational algebra has developed various algorithms for processing polynomials, including polynomial system solving, or more generally, Gröbner basis computation of an associated ideal. In this talk, I present a new paradigm for addressing such problems, i.e., a machine-learning approach using a Transformer. The learning approach does not require an explicit algorithm design and can return the solutions in (roughly) constant time. This approach does not necessarily return correct solutions but can be a practical compromise against large-scale problems for which math algorithms run into a timeout. I’ll show several successful cases and challenges particular to this approach.
Matthias Köppe
SageMath is a major integrating force in the mathematical software world that provides convenient and unified access to the best libraries and systems for polyhedral geometry, mixed integer linear programming, lattices, graph theory, commutative algebra, symbolic computation, differential geometry, computational group theory, and much more. Long developed as a monolithic, closed system, a multi-year modularization project has prepared it for a new role as providing reusable, pip-installable libraries for the varied mathematical needs of the DOMLverse.
Leo Liberti
Given an integer $K>0$ and a simple undirected edge-weighted graph $G=(V,E,d)$, the Distance Geometry Problem (DGP) asks to find a realization of $G$ such that vertices are points in $\mathbb R^K$ and edges ${i,j}$ are segments with the same length as the corresponding edge weights. The unassigned DGP (uDGP) replaces the graph $G$ with a list of positive values $L$. In other words, it asks to find an assignment that associates to each value in $L$ a vertex pair ${i,j}$ which must be realized as a segment with endpoints $i,j$ and length equal to the corresponding edge weigth. In this talk we discuss motivating applications, some Mathematical Programming formulations, and preliminary results.
Bruno Lourenço
Hyperbolicity cones are a family of cones that include, in particular, the PSD matrices. However, in contrast to PSD matrices, the projection operator does not have a closed form in general. In this talk we discuss some theoretical results and a numerical approach based on the Frank-Wolfe method for computing the projection onto arbitrary hyperbolicity cones. We will also show numerical experiments where the cones come from hyperbolic polynomials with millions of monomials. This is a joint work with Takayuki Nagano and Akiko Takeda.
Pierre-Louis Poirion
In this talk, we consider an optimization problem over the Stiefel manifold. We will propose an iterative algorithm that consider, at each iteration, a function restricted to a small random sub-manifold on which retractions can be performed at a small cost. We will prove convergence of our method and illustrate the results numerically.
Sebastian Pokutta
We present two novel six-colorings of the Euclidean plane that avoid monochromatic pairs of points at unit distance in five colors and monochromatic pairs at another specified distance $d$ in the sixth color. Such colorings have previously been known to exist for $0.41 < \sqrt{2} - 1 \leq d \leq 1/\sqrt{5} < 0.45$. Our results significantly expand that range to $0.354 \leq d \leq 0.657$, the first improvement in 30 years. Notably, the constructions underlying this were derived by formalizing colorings suggested by a custom machine learning approach.
Ting Kei Pong
In this talk, we consider a class of L1-regularized optimization problems and the associated smooth “over-parameterized” optimization problems built upon the Hadamard difference parametrization (HDP). We show that second-order stationary points of the HDP-based model correspond to some stationary points of the corresponding L1-regularized model. More importantly, we show that the Kurdyka-Łojasiewicz (KL) exponent of the HDP-based model at a second-order stationary point can be inferred from that of the corresponding L1-regularized model under suitable assumptions. Our assumptions are general enough to cover a wide variety of loss functions commonly used in L1-regularizations, such as the least squares loss function and the logistic loss function. We also discuss how these KL exponents can help deduce the local convergence rate of a standard gradient method for minimizing the HDP-based models.
Vera Roshchina
I will review some known results about the facial structure of convex sets that are specific to the infinite-dimensional setting, both in general vector spaces and in topological spaces where different notions of relative interior can be considered. I will also present a new notion called “face relative interior” that relies on topology, but is grounded in facial structure, and introduce some of its properties and calculus rules. The talk is based on joint work with Reinier Díaz Millán.
Jan-Dirk Schmöcker
Route choice in networks with uncertainties leads to the problem of optimal hyperpaths. The size of the hyperpath can reduce or enlarge depending on fares. This will be discussed as well as the concept of a Delay Insurance Fares problem: A transport service provider offers a premium service that provides preferential treatment and needs to consider capacities and pricing of the service. The talk will conclude with some remarks on practical applications as well as new big data source and machine learning for travel demand forecasting.
Renata Sotirov
The complex cut polytope is the convex hull of Hermitian rank one matrices $xx^H$, where the elements of $x \in \mathbb{C}^n$ are $m$-th unit roots. For $m=2$, the complex cut polytope corresponds to the well-known cut polytope. We first introduce the complex elliptope that is considered to be the first semidefinite lifting of the complex cut polytope. Then, we show how to derive valid cuts in the complex plane that separate the complex elliptope from the complex cut polytope. Further, we consider a second semidefinite lifting of the complex cut polytope for $m=\infty$. This lifting is equivalent to the complex Lasserre-type liftings of the same order from the literature, while being of smaller size. Finally, we present numerical results that verify quality of our complex semidefinite approximations of the complex cut polytope.
Masashi Sugiyama
When solving a classification problem with deep learning, it is not difficult to achieve perfect classification for training data (i.e., zero training error). On the other hand, in many practical classification problems, the minimum achievable test error (i.e., the Bayes error) is not zero. In this talk, we discuss two topics regarding the training error and Bayes error in deep learning: (i) Do we need to aim for zero training loss (computed by a surrogate loss such as the cross-entropy loss) after achieving zero training error (computed by the zero-one loss)? (ii) Can we estimate the Bayes error accurately? Based on joint works with Ishida et al.
Akiko Takeda
Random projection techniques based on the Johnson-Lindenstrauss lemma are used for randomly aggregating the constraints or variables of optimization problems while approximately preserving their optimal values, which leads to smaller-scale optimization problems. In this talk, we show a few applications of random matrix techniques for constructing random subspace algorithms that iteratively solve smaller-scale subproblems and discuss their convergence speed. This talk is based on joint works with Ryota Nozawa, and Pierre-Louis Poirion.
Takashi Tsuchiya
Analysis of positve factors for development of countries is one of the important issues in the world. In this talk, we analyze relation between development of countries and autonomy of political systems of 193 countries over the period of 1990 to 2022 using a Markov model, where Human Development Index by United Nations Development Programme is employed as development index and Freedom House Score by Freedom House is employed as index for political autonomy. We demonstrate plausibility of the model and discuss its implication from various point of view. This is a joint work with Abigail Adomaco Boadi.
Takashi Ui
An equilibrium outcome in an incomplete information game depends not only on the payoff structure but also on the information structure. Information design characterizes an optimal information structure that maximizes a given objective function under the constraint that agents follow an equilibrium. This paper addresses information design with linear best responses of agents, quadratic objective functions, and payoff states distributed according to Gaussian distributions. We formulate the problem as semidefinite programming (SDP) and use the duality principle to characterize an optimal information structure. A Gaussian information structure is shown to be optimal among all information structures. A necessary and sufficient condition for optimality is that the covariance matrix of actions and states satisfies linear constraints derived from the primal and dual SDP, or equivalently, the realizations of actions and states satisfy the corresponding linear constraints. As a result, an observed action profile typically reveals the true state even if individual agents have only partial knowledge. In symmetric network games, an optimal information structure inherits this symmetry, which facilitates its computation. Based on joint works with Masaki Miyashita.
Akiko Yoshise
Machine learning technologies rely on high-quality, diverse datasets for predictive power and generalizability. However, integrating data from multiple sources introduces significant privacy challenges due to global regulations like GDPR. We present the Procrustean Data Collaboration framework to address this, combining the Orthogonal Procrustes Problem with the Privacy-Preserving Data Collaboration Analysis method. This framework leverages the irreversibility of linear dimensionality reduction inherent to Data Collaboration Analysis for privacy protection while providing theoretical and empirical improvements via the Orthogonal Procrustean formulation.
Andrea Walther
The retail industry is governed by crucial decisions on inventory management, discount offers and stock clearings yielding various optimization problems. One important task is the prediction of the demand (sales) elasticity with respect to product prices. Another one is the dynamic revenue maximization problem, which takes in the coefficients of demand as inputs. While both tasks present nonsmooth optimization problems, the latter is a challenging nonlinear problem in massive dimensions that is also subject to constraints. Traditional approaches to solve such problems relied on using reformulations and approximations, thereby leading to potentially suboptimal solutions. In this work, we exploit the nonsmooth structure generated by the piecewise linear and piecewise smooth structure of the target function. For this purpose, we present an adaptation of the Constrained Active Signature Method (CASM). Two real world retail examples (UK and US market data from 2017-2019) and one simulated use-case are studied from an empirical standpoint. Numerical results demonstrate good performance of our approach.
Yuan Zhou
The study of triangulations of finite point configurations is a rich topic at the intersection of combinatorics, geometry, and optimization. It is well known that two point configurations $A$ and $B$ with identical oriented matroids (encoded by the set of circuits or cocircuits, equivalently) have the same set of triangulations. Given a triangulation $T$ of $A$, we consider the coordinates of the points in $A$ as parameters, and ask: which set of polynomial inequalities on the parameters ensures that $T$ is a triangulation of $A$? That is, we are interested in the conditions under which $A$ and $B$ share a particular triangulation but not necessarily all triangulations. We show that, in low dimensions, a restricted set of cocircuits corresponding to hyperplanes spanned by facets of the simplices in $T$ suffices. This gives convenient parametric volume formulas with few side conditions, with numerous obvious applications in cutting planes, computational biology and optimal control.
Previous Editions
[ DO x ML 2018 | DO x ML 2019 | DO x ML 2020 | DO x ML 2021 | DO x ML 2023 ]