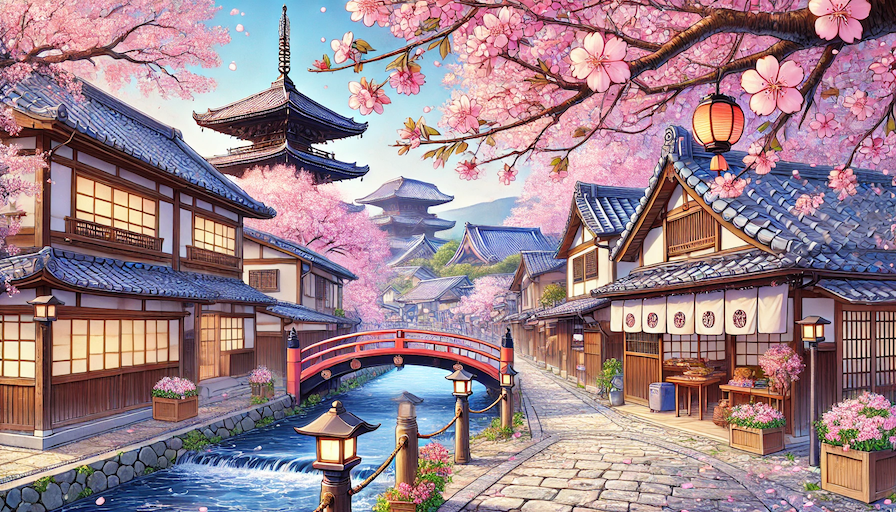
Seventh Conference on
Discrete Optimization and Machine Learning
Spring 2025 x Kyoto, Japan
Coordinates
Dates: 12th - 16th May, 2025
Place: Research Institute for Mathematical Sciences (RIMS), Kyoto, Japan
Organizers: Antoine Deza, Kazuhisa Makino, Sebastian Pokutta, Akiyoshi Shioura
Within the 2025 RIMS Project
Advances in Theoretical Research on Mathematical Optimization
Conference Dinner: May 13 at 6 pm at Camphora (Kyoto University Campus Restaurant)
Yoshidahonmachi, Sakyo Ward, Kyoto, 606-8317, Japan. Located near main gate [map]
Accomodation
Hotel recommendations for Kyoto. Note that it is important to book early.
Participants
- Amir Ali Ahmadi
- David Avis
- Mourad Baïou
- Chenglong Bao
- David Bremner
- Hong Chu
- Claudia D'Ambrosio
- Antoine Deza
- Daniel Deza
- Ricardo Fukasawa
- Elisabeth Gaar
- Dan Garber
- Patrick Gelß
- Lukas Glomb
- Andrés Gómez
- Adrian Göß
- Swati Gupta
- Akshay Gupte
- Nir Halman
- Deborah Hendrych
- Ayumi Igarashi
- Michael Joswig
- Ken Kobayashi
- Michel de Lara
- Jon Lee
- Weizi Li
- Leo Liberti
- Andrew Lim
- Bruno Lourenço
- Zhi-Quan Luo
- Kazuhisa Makino
- David Martínez-Rubio
- Frédéric Meunier
- Gioni Mexi
- Konrad Mundiger
- Sai Ganesh Nagarajan
- Bento Natura
- Frank Nielsen
- Axel Parmentier
- Dmitrii Pasechnik
- Marc Pfetsch
- Pierre-Louis Poirion
- Sebastian Pokutta
- Ting Kei Pong
- Lionel Pournin
- Christophe Roux
- Hiroyuki Sakai
- Lars Schewe
- Jan-Dirk Schmöcker
- Christof Schütte
- Akiyoshi Shioura
- Martin Skutella
- Christoph Spiegel
- Bartolomeo Stellato
- Tamon Stephen
- Masashi Sugiyama
- Sri Harshitha Tadinada
- Kim-Chuan Toh
- Berkant Turan
- Andrea Walther
- Ailing Xie
- Yao Xie
- Haoxiang Yang
- Yuan Zhou
- Max Zimmer
- Yuriy Zinchenko
Program
May 12
Time | Speaker | Talk Title (for abstract click title or see below) |
---|---|---|
09:30 - | Registration/Coffee | |
10:00 - | Session Chair: TBD | |
10:00 - | Marc Pfetsch |
Combinatorial Aspects of Physical Networks
|
10:30 - | Yao Xie |
Variable selection for kernel two-sample tests.
|
11:00 - | David Martínez-Rubio |
Convergence and Trade-Offs in Riemannian Gradient Descent and Proximal Point
|
11:30 - | Frédéric Meunier |
The Lemke—Howson algorithm for oriented matroids, and applications
|
Lunch Break | ||
13:30 - | Session Chair: TBD | |
13:30 - | Amir Ali Ahmadi |
Disjunctive Sum of Squares
|
14:00 - | Ricardo Fukasawa |
Game-theoretic based relaxations in bilevel optimization
|
14:30 - | Michael Joswig |
Hypersimplices, tropical geometry and finite metric spaces
|
Coffee Break | ||
15:30 - | Session Chair: TBD | |
15:30 - | Chenglong Bao |
Convergence Analysis of Two Stochastic Algorithms
|
16:00 - | Andrea Walther |
On a Frank-Wolfe method for convex piecewise smooth functions
|
16:30 - | Leo Liberti |
Computation with distance geometry
|
May 13
Time | Speaker | Talk Title (for abstract click title or see below) |
---|---|---|
09:30 - | Coffee | |
10:00 - | Session Chair: TBD | |
10:00 - | Masashi Sugiyama |
Classification from Wrongly Labeled Data: Recent Advances in Complementary Classification
|
10:30 - | Dan Garber |
A Linearly Convergent Frank-Wolfe-type Method for Smooth Convex Minimization over the Spectrahedron
|
11:00 - | Lars Schewe | Discrete Optimization for better outage planning of electricity networks |
11:30 - | Mourad Baïou |
Game theory models and algorithms for trading demands
|
Lunch Break | ||
13:30 - | Session Chair: TBD | |
13:30 - | Andrés Gómez |
Real-time solutions for a class of mixed-integer optimization problems
|
14:00 - | Claudia D'Ambrosio |
Surrogate mixed integer nonlinear models by means of regression splines
|
14:30 - | Axel Parmentier |
Primal Dual Algorithm for Empirical Risk Minimization in Combinatorial Optimization Augmented Learning
|
Coffee Break | ||
15:30 - | Session Chair: TBD | |
15:30 - | Akshay Gupte |
Machine learning methods for finding good solutions to nonconvex quadratic and multiobjective optimization
|
16:00 - | Tamon Stephen |
Hypergraph Transversal Pairs Near the Fredman-Khachiyan Bound
|
16:30 - | Nir Halman |
Packing Squares independently
|
Conference Dinner at Camphora |
May 14
Time | Speaker | Talk Title (for abstract click title or see below) |
---|---|---|
09:30 - | Coffee | |
10:00 - | Session Chair: TBD | |
10:00 - | Zhi-Quan Luo |
Finite Horizon Optimization
|
10:30 - | Kim-Chuan Toh |
A Low-Rank ALM for Doubly Nonnegative Relaxations of Mixed-Binary QP
|
11:00 - | Pierre-Louis Poirion |
Efficient Optimization with orthogonality constraints
|
11:30 - | David Bremner |
Optimizing over the union of linear subspaces
|
Lunch Break | ||
13:30 - | Session Chair: TBD | |
13:30 - | Martin Skutella |
Complexity of Injectivity and Verification of ReLU Neural Networks
|
14:00 - | Lionel Pournin |
The expansion of half-integral zonotopes
|
14:30 - | Bento Natura |
Near-Optimal Path Following in the $\ell_2$-Neighborhood
|
Coffee Break | ||
15:30 - | Poster Session |
May 15
Time | Speaker | Talk Title (for abstract click title or see below) |
---|---|---|
09:30 - | Coffee | |
10:00 - | Session Chair: TBD | |
10:00 - | Swati Gupta |
Bridging Discrete and Continuous Perspectives in Combinatorial Polytopes
|
10:30 - | Bartolomeo Stellato |
Data-driven analysis and design of convex optimization algorithms
|
11:00 - | Haoxiang Yang |
Learning to Generate Lagrangian Cuts
|
11:30 - | Andrew Lim |
The exploration, exploitation robustness tradeoff
|
Lunch Break | ||
13:30 - | Session Chair: TBD | |
13:30 - | Dmitrii Pasechnik |
Harmonic moment problem on polyhedra
|
14:00 - | Ken Kobayashi |
Learning decision trees and forests with algorithmic recourse
|
14:30 - | Christoph Spiegel |
Neural Discovery in Mathematics
|
Coffee Break | ||
15:30 - | Session Chair: TBD | |
15:30 - | Jan-Dirk Schmöcker |
Tourist Travel Demand Management and Novel Public Transport Pricing Strategies
|
16:00 - | Yuriy Zinchenko |
Can an infeasible MIP solve itself?
|
16:30 - | Yuan Zhou | TBD |
May 16
Time | Speaker | Talk Title (for abstract click title or see below) |
---|---|---|
09:30 - | Coffee | |
10:00 - | Session Chair: TBD | |
10:00 - | Jon Lee |
ADMM for convex optimization problems associated with statistical models
|
10:30 - | Christof Schütte | Cheeger constants, transfer operators and transport networks |
11:00 - | Frank Nielsen |
Divergences and maximal invariants
|
11:30 - | Michel de Lara |
Geometry of Sparsity-Inducing Norms
|
Lunch Break | ||
13:30 - | Session Chair: TBD | |
13:30 - | Ting Kei Pong |
A single-loop proximal-conditional-gradient penalty method
|
14:00 - | Sai Ganesh Nagarajan |
Algorithms and Complexity for Two-Team Polymatrix Zero-Sum Games: Revisiting Constrained Bilinear Minmax Optimization
|
14:30 - | Bruno Lourenço |
Faces of homogeneous convex cones and applications to homogeneous chordal PSD matrices
|
Abstracts
Amir Ali Ahmadi
We introduce the concept of “disjunctive sum of squares” for certifying nonnegativity of polynomials. Unlike the popular sum of squares approach where nonnegativity is certified by a single algebraic identity, the disjunctive sum of squares approach certifies nonnegativity with multiple algebraic identities. Our main result is a disjunctive Positivstellensatz proving that we can keep the degree of each algebraic identity as low as the degree of the polynomial whose nonnegativity is in question. Based on this result, we construct a semidefinite programming based converging hierarchy of lower bounds for the problem of minimizing a polynomial over a compact basic semialgebraic set, where the size of the largest semidefinite constraint is fixed throughout the hierarchy. We further prove a second disjunctive Positivstellensatz which leads to an optimization-free hierarchy for polynomial optimization. We specialize this result to the problem of proving copositivity of matrices. Finally, we describe how the disjunctive sum of squares approach can be combined with a branch-and-bound algorithm and we present numerical experiments on polynomial, copositive, and combinatorial optimization problems. Joint work with Sanjeeb Dash (IBM), Yixuan Hua (Princeton), and Bartolomeo Stellato (Princeton).
Mourad Baïou
We introduce a new cooperative game theory model that we call production-distribution game. It models efficient sharing principles for practical collaboration in transportation. The originality of our model lies in the fact that the value/strength of a player does not only depend on the individual cost or benefit of the goods she owns but also on her market shares (customers demand). We prove that we can compute the nucleolus efficiently, in a nontrivial, interesting special case. We provide two algorithms to compute the nucleolus: a simple separation algorithm and a fast primal-dual one. We also show that our results can be used to tackle more general versions of the problem.
Chenglong Bao
In this talk, we will explore convergence analysis for two stochastic optimization algorithms. First, we establish a tight convergence rate for the stochastic proximal point algorithm in solving stochastic composite optimization problems. Second, we present global convergence results for the stochastic gradient descent algorithm with adaptive noise injection, tailored for minimizing nearly convex functions.
David Bremner
We compare some different approaches for cut generation in integer optimization when the feasible region is the intersection of a convex polytope and the (non-convex) union of low dimensional linear subspaces. This arises as a sub-problem in optimizing under symmetry, but is also of potentially more general application.
Claudia D'Ambrosio
Complex phenomena can be accurately described by means of data-driven mathematical models. However, being able to integrate these models within a mathematical optimization framework can be, in general, very challenging. In fact, many of these data-driven models are `black-box’, in the sense that they do not have an explicit mathematical formula which describes them. In other cases, even if an explicit expression exists, including it into a mathematical optimization model may make solving the problem computationally intractable. We propose to use a special kind of surrogate models, regression splines, to deal with functions of this kind which appear in Mixed Integer Nonlinear Programming (MINLP) problems. The choice of spline functions is not arbitrary. On one hand, they offer a good compromise between accuracy and complexity. On the other hand, their functional form allows us to exploit separability and approximate general non-convex MINLPs by a more tractable subclass of problems.
Ricardo Fukasawa
We introduce a framework for relaxing Stackelberg zero-sum games with perfect information, with a focus on those arising from discrete bilevel optimization. The framework consists of operators which transform games while maintaining an approximation or bound on the optimal objective value. This simplifies and generalizes our two previous works on knapsack and matroid interdiction. It also generalizes well-known techniques including the high point relaxation and the derivation of bounds by restricting/relaxing the lower level problem. To further motivate this approach, we apply it to matching interdiction, in which the objective is to interdict (i.e., remove) edges from a graph so that the maximum matching weight is minimized, subject to a knapsack constraint on the set of interdicted edges. We present a branch-and-bound algorithm for this problem which uses a new lower bound derived using our framework.
Dan Garber
We consider the problem of minimizing a smooth and convex function over the n-dimensional spectrahedron — the set of real symmetric n×n positive semidefinite matrices with unit trace, which underlies numerous applications in statistics, machine learning and additional domains. Standard first-order methods often require high-rank matrix computations which are prohibitive when the dimension n is large. The well-known Frank-Wolfe method on the other hand, only requires efficient rank-one matrix computations, however suffers from worst-case slow convergence, even under conditions that enable linear convergence rates for standard methods. In this work we present the first Frank-Wolfe-based algorithm that only applies efficient rank-one matrix computations and, assuming quadratic growth and strict complementarity conditions, is guaranteed, after a finite number of iterations, to converges linearly, in expectation, and independently of the ambient dimension.
Andrés Gómez
We consider mixed-integer quadratic optimization problems with banded matrices and indicator variables. These problems arise pervasively in statistical inference problems with time-series data, where the banded matrix captures the temporal relationship of the underlying process. In particular, the problem studied arises in monitoring problems, where the decision-maker wants to detect changes or anomalies. We propose to solve these problems using decision diagrams. In particular we show how to exploit the temporal dependencies to construct diagrams with size polynomial in the number of decision variables. We also describe how to construct the convex hull of the set under study from the decision diagrams, and how to deploy the method online to solve the problems in milliseconds via a shortest path algorithm.
Adrian Göß
Practical decision-making problems such as in supply chain management or resource allocation, typically require the selection of particular integer values with nonlinear interrelationships. The resulting problem class of mixed-integer nonlinear programming (MINLP) is considered challenging and requires a careful balance between computation time and accuracy. Recent approaches like the GBM method aim to address this challenge by leveraging advances in the more specific class of mixed-integer quadratically-constrained programming (MIQCP) via parabolic approximations. The theoretical guarantees on the approximation quality of the GBM method are valid for arbitrary finite dimension, but require extensive computational overhead in practice. We present an algorithm that provides the required paraboloids for one dimensional constraint functions in a fraction of the time needed by the original method and test the returned approximation by tackling a subset of the MINLPLib instances. The core of the algorithm computes one paraboloid at a time, relying on Newton’s method and the solution of small 3x3 equality systems only. We provide theoretical as well as computational evidence of the algorithm’s functionality. The ability to provide good approximate solutions to MINLP problems (from MINLPLib) is showcased by substituting nonlinear constraints with their parabolic approximations and solving the resulting MIQCP. We see an extensive increase in computational efficiency in comparison to the original GBM method for one dimensional functions. The results on MINLPLib instances indicate the potential of solving MINLP problems by reducing them to MIQCP ones.
Swati Gupta
There are significant gaps in our knowledge when it comes to convex optimization over combinatorial polytopes, due to the evolution of the theory of discrete algorithms being largely independent compared to general continuous optimization methods. In this talk, I will discuss some recent work in bridging this gap, by studying feasible directions of descent for constrained minimization, and revisiting the oracles used in first-order methods.
Akshay Gupte
We consider machine learning methods for selecting optimal parameters for two classes of challenging optimization problems – nonconvex quadratically constrained quadratic programs, and multiobjective mixed integer linear optimization. For both problems we are interested in finding good quality feasible solutions. For QCQPs, we consider adaptive MILP discretizations that were recently developed but for which the parameters governing the discretization are selected and updated in some ad-hoc manner. For the second, we are interested in Pareto solutions that are spread wide apart on the efficient frontier, and can be generated through different (equivalent) scalarizations, each with its own set of parameters.
Nir Halman
Given a set of squares and a strip of bounded width and infinite height, we consider a square strip packaging problem, which we call the square independent packing problem (SIPP), to minimize the strip height so that all the squares are packed into independent cells separated by horizontal and vertical partitions. For the SIPP, we first investigate efficient solution representations and propose a compact representation that reduces the search space from \(O(n!)\) to \(O(2^n)\), with \(n\) the number of given squares, while guaranteeing that there exists a solution representation that corresponds to an optimal solution. Based on the solution representation, we show that the problem is NP-hard. To solve the SIPP, we propose a dynamic programming method that can be extended to a fully polynomial-time approximation scheme (FPTAS). We also propose three mathematical programming formulations based on different solution representations and confirm the performance of these algorithms through computational experiments. Finally, we discuss several extensions that are relevant to practical applications.
Michael Joswig
The hypersimplex \(\Delta(k,n)\) is the convex polytope which is the set of those points in the unit cube \([0,1]^n\) which satisfy the affine linear equation \(\sum x_i=k\). We report on known and new results concerning the secondary fan \(\Sigma(k, n)\), which stratifies the regular subdivisions of the \(\Delta(k,n)\). For tropical geometry this is relevant because \(\Sigma(k,n)\) contains the tropical Grassmannian \({\rm TGr}(k,n)\) as a subset; we discuss that relationship. Furthermore, in the special case \(k = 2\) the fan \(\Sigma(2,n)\) is closely related to the metric fan \({\rm MF}(n)\), which forms a natural parameter space for the metric spaces on \(n\) points. Our analysis of the fans \(\Sigma(2,n)\) improves known results of Bandelt and Dress concerning structural properties of finite metric spaces, with applications to phylogenetics. The new results are joint work with Laura Casabella and Lars Kastner.
Ken Kobayashi
This talk proposes a new algorithm for learning accurate tree-based models while ensuring the existence of recourse actions. Algorithmic Recourse (AR) aims to provide a recourse action for altering the undesired prediction result given by a model. Typical AR methods provide a reasonable action by solving an optimization task of minimizing the required effort among executable actions. However, such actions do not always exist for models optimized only for predictive performance. In this study, we formulate the task of learning an accurate classification tree to ensure reasonable actions exist for as many instances as possible. Then, we propose an efficient top-down greedy algorithm by leveraging the adversarial training techniques. We also show that our proposed algorithm can be applied to the random forest, which is known as a popular framework for learning tree ensembles.
Michel de Lara
Sparse optimization seeks an optimal solution with few nonzero entries. To achieve this, it is common to add to the criterion a penalty term proportional to the $\ell_1$-norm, which is recognized as the archetype of sparsity-inducing norms. In this approach, the number of nonzero entries is not controlled a priori. By contrast, in this talk, we focus on finding an optimal solution with at most $k$ nonzero coordinates (in short, $k$-sparse vectors), where $k$ is a given sparsity level/budget. For this purpose, we study the class of generalized $k$-support norms, induced by a given source norm. When added as a penalty term, we provide conditions under which such generalized $k$-support norms promote $k$-sparse solutions. The result follows from an analysis of the exposed faces of closed convex sets generated by $k$-sparse vectors, and of how primal support identification can be deduced from dual information. Finally, we provide geometric properties of the unit balls for the $k$-support norms and their dual norms when the source norms belong to the $\ell_p$-norms family. Joint work with Jean-Philippe Chancelier, Antoine Deza, and Lionel Pournin.
Jon Lee
We consider a few key statistical problems, which can be profitably relaxed to convex-optimization problems in rather subtle ways. We investigate the use of various ADMM (the alternating directions method of multipliers) algorithms to enable the solution of large instances. The problems that we look at are MESP (maximum-entropy sampling problem), D-Optimality (for regression design), and the calculation of sparse generalized inverses (with application to calculating sparse universal least-squares solvers). This talk covers various joint works with Marcia Fampa, Gabriel Ponte and Luke Xu.
Leo Liberti
We exploit polynomial reductions from binary linear programming (BLP) to satisfiability (SAT), from SAT to 3SAT, and from 3SAT to the distance geometry problem (DGP), which is a problem having continuous variables describing vectors in a Euclidean space of given dimension. By means of the reduction, we can solve binary optimization using continuous methods for solving the DGP. We discuss theoretical issues and computational features of this unusual solution process.
Andrew Lim
Parameter uncertainty and model misspecification are fundamental concerns in multi-period data-driven decision making, but little is understood about the tradeoff between exploration, exploitation and robustness. To study this tradeoff, we consider a robust optimal stopping problem with Bayesian learning. We show that an ambiguity-averse decision maker spends less time exploring, even though model uncertainty is reduced by having more data, because the ``learning shock” for each posterior update increases the sensitivity of the expected profit to model misspecification. We also show that a willingness to explore can be restored by introducing a hedging instrument that offsets the learning shock.
Bruno Lourenço
A regular convex cone is homogeneous if its automorphism group acts transitively on its interior. Notably, if we have a chordal graph G that is “4-path free”, then the cone of PSD matrices with sparsity pattern given by G is homogeneous. In this case, we say that G is homogeneous chordal. In this talk, we discuss some recent results on the faces of homogeneous convex cones and discuss its applications to PSD matrices where the sparsity pattern is given by a homogeneous chordal graph.
Zhi-Quan Luo
In practical scenarios, there is often a strict upper bound on the number of algorithm iterations that can be performed within a given time limit. This raises the question of optimal step size and hyperparameter design for a fixed iteration budget. We present recent advances in effectively addressing this highly non-convex problem for gradient descent and other algorithms. Additionally, we extend the DeepMind work on AlphaTensor and introduce new reductions in the number of operations required for computation of more general matrix expressions. This provides further acceleration of calculations in linear algebra.
David Martínez-Rubio
Convergence analyses of the two most fundamental algorithms for geodesically convex optimization were incomplete until our work. We design techniques and develop new full analyses that reveal some trade-off between geometric, iterate boundedness and computational efficiency. As a consequence, we can also obtain minmax methods for geodesically convex concave without knowing the initial distance to a saddle point for the first time.
Frédéric Meunier
Lemke and Howson proposed in 1964 a pivot-based algorithm for solving bimatrix games. The year after, Lemke proposed a closely related algorithm for solving a large class of linear complementarity problems. Although Todd extended the Lemke algorithm to oriented matroids in 1984, the extension of the Lemke—Howson algorithm to oriented matroids has not yet been addressed in the literature. Actually, achieving such an extension requires solving a few issues. One of them is to propose a relevant definition of Nash equilibria for oriented matroids. Another one is more challenging, namely finding the counterpart of the following preliminary operation of the classical Lemke—Howson algorithm: the translation of the payoff matrices so as to make them positive. In this work, we show how to overcome these challenges and extend the Lemke—Howson algorithm to oriented matroids. A few applications are discussed, such as a strengthening of a theorem by McLennan and Tourky, a combinatorial interpretation of the orientation of the pivot step in the classical version, and the localization of tropical bimatrix games in the class PPAD. Joint work with Xavier Allamigeon and Stéphane Gaubert.
Sai Ganesh Nagarajan
Adversarial multiplayer games are an important object of study in multiagent learning. In particular, polymatrix zero-sum games are a multiplayer setting where Nash equilibria are known to be efficiently computable. Towards understanding the limits of tractability in polymatrix games, we study the computation of Nash equilibria in such games where each pair of players plays either a zero-sum or a coordination game. A setting that is particularly interesting is where players can be grouped into a small number of teams of identical interest [Von Stengel and Koller 1997]. While the three-team version of the problem is known to be PPAD-complete, the complexity for two teams has remained open [Cai and Daskalakis 2011]. In this talk, we show that the two-team version is CLS-hard and we do so by proving the hardness of finding any stationary point in the simplest type of non-convex-concave min-max constrained optimization problem, namely for a class of bilinear polynomial objective functions. Finally, we discuss some algorithms for a restricted version of this problem, namely a team with non-interacting adversaries.
Bento Natura
We investigate a variant of the trust region interior point method (TR-IPM) which was originally given by Lan, Monteiro and Tsuchiya (SIAM J. Optim. 2009). We show that the iteration complexity of this algorithm is essentially optimal in the $\ell_2$-neighbourhood of the central path. Namely, the iteration complexity of the TR-IPM is upper bounded by the minimum number of segments of any piecewise linear curve traversing in a close $\ell_2$-neighborhood of the central path. This strengthens the previous bounds that were in terms of the Dikin-Todd-Stewart conditional number of the constraint matrix. In particular, it improves the universal bound given by Allamigeon et al. (SIAM J. Comput. 2025) by $O(n^{1.5}\log n)$ when in the $\ell_2$-neighborhood. In particular, it matches the iteration complexity of any such existing IPMs which also have direct dependence on the problem dimension and the Dikin-Todd-Stewart conditional number of the constraint matrix. Lan, Monteiro and Tsuchiya only gave a weakly polynomial algorithm for solving the trust region problem, and left it as an open question to develop a strongly polynomial algorithm. We resolve this question by presenting a new strongly polynomial algorithm that solves a parametric minimum norm problem to a very high accuracy. As a result, each iteration of our TR-IPM can be implemented in strongly polynomial time. Our algorithm for the parametric problem relies on approximately computing the singular value decomposition of an associated matrix. These singular values enable us to locate the optimal parameter value in a narrow interval, where Newton’s method exhibits quadratic convergence. Joint work with Daniel Dadush, Haoyuan Ma, and László A. Végh.
Frank Nielsen
In this talk, we first start by presenting Bregman divergences which stems from the optimization community and gained huge popularity in machine learning thanks to a remarkable identity with the relative entropy between exponential family densities. We explain more generally how to reconstruct statistical divergences from Bregman divergences when considering various convex generators induced by exponential families. Second, we describe the concept of maximal invariance under a group action and show how it yields structural results on information-theoretic divergences for several statistical models.
Axel Parmentier
As industry seeks to make its processes more resilient, data driven optimization is gaining momentum in Operation Research. Resilience involves managing uncertainty when optimizing supply chains, while efficiency requires scalable combinatorial optimization algorithms, as most gains from operations research algorithms come from decreasing marginal costs. This underscores the need for scalable algorithms for combinatorial and stochastic optimization problems, whether they are dynamic, tactical, or strategic. Policies encoded as neural networks with a combinatorial optimization layer have demonstrated their efficiency in these settings when trained in a decision-focused manner. Until now, such policies have been trained using supervised learning, often based on Fenchel-Young losses, and considered as heuristics. We instead focus on risk minimization. By leveraging the connection between Fenchel-Young losses and Bregman divergences, we introduce a deep learning-compatible primal-dual algorithm and show that it converges to the optimum of the empirical risk minimization problem in some settings. This new approach has two advantages. First, numerical experiments show that it learns better policies. Second, we prove some generalization properties that provide upper bounds on the optimality gap, which hold in expectation.
Dmitrii Pasechnik
Classical harmonic polynomials are the polynomial solutions $f$ of the Laplace PDE $(\sum_k \partial^2/\partial x_k^2)f(x_1,\ldots,x_n)=0$; alternatively, they can be indentified with the quotient ring $\mathbb{R}[x]/(\sum_k x_k^2)\mathbb{R}[x]$. Harmonic functions arise in a number of natural settings in science and engineering. Moments of a measure $\mu$ w.r.t. to a family of test functions ${f_K}$ are integrals $\mu_K = \int f_K(x) d\mu(x)$. Determining $\mu$ from the multi-sequence ${\mu_K}$ is called the moment problem (w.r.t. to ${f_K}$). While often $\mu$ is determined uniquely by ${f_K}$, allowing a convenient representation of $\mu$ for various problems, such as optimisation, this is not always the case for $f_K$ being harmonic polynomials—such examples are already known for $n=2$ and $\mu$ the uniform measure on a polygon - the case studied in some detail by Pasechnik and Shapiro (2014). In this talk we deal with the case $n>2$.
Marc Pfetsch
This talk considers combinatorial properties of physical networks like water, gas, heat, and electrical networks. One common model is given by potential-based flows, for which, in general, the corresponding flows depend in a nonlinear way on potentials (e.g., pressures) and are unique. The talks will highlight the interplay between the nonlinear behavior and combinatorial decisions, e.g., the fact that flows are acyclic in this model. We fill focus on topology optimization of such networks. Here, valid inequalities on the binary decision variables are derived using the nonlinear behavior of the network.
Pierre-Louis Poirion
Optimization with orthogonality constraints frequently arises in machine learning. Riemannian optimization offers a powerful framework for solving these problems by equipping the constraint set with a Riemannian manifold structure and performing optimization intrinsically on the manifold. This approach typically involves computing a search direction in the tangent space and updating variables via a retraction operation. However, as the size of the variables increases, the computational cost of the retraction can become prohibitively high, limiting the applicability of Riemannian optimization to large-scale problems. To address this challenge and enhance scalability, we propose a novel approach that restricts each update on a random submanifold, thereby significantly reducing the per-iteration complexity. We introduce two sampling strategies for selecting the random submanifolds and theoretically analyze the convergence of the proposed methods. We provide convergence results for general nonconvex functions and functions that satisfy Riemannian Polyak-Łojasiewicz condition as well as for stochastic optimization settings. Additionally, we demonstrate how our approach can be generalized to quotient manifolds derived from the orthogonal manifold. Extensive experiments verify the benefits of the proposed method, across a wide variety of problems.
Ting Kei Pong
We consider the problem of minimizing the separable sum of two proper closed convex functions over a linear coupling constraint. We assume that one of these functions is prox-friendly, while the other function admits efficient (generalized) linear oracles. We propose a single-loop variant of the standard penalty method for this problem, where in each iteration, we successively perform one proximal-gradient step and one conditional-gradient step on the quadratic penalty function, followed by an update of the penalty parameter via an explicit formula. We derive iteration complexity bound and local convergence rate under standard constraint qualifications and Kurdyka-Łojasiewicz assumptions. We illustrate numerically the convergence behavior of our algorithm on minimizing the $L_1$ norm subject to a residual error measured by $L_p$ norm, $1 < p < 2$. This is a joint work with Liaoyuan Zeng and Hao Zhang.
Lionel Pournin
The expansion of a graph G is a parameter that allows to analyse random walks on G. This talk is about the expansion of the edge-graphs of certain polytopes. A conjecture of Milena Mihail and Umesh Vazirani states that the edge-graph of every 0/1-polytope has expansion at least $1$. We will show that the generalisation of this conjecture to half-integral polytopes does not hold by constructing $d$-dimensional half-integral polytopes whose expansion decreases exponentially fast with $d$. If one restricts the analysis to half-integral zonotopes, a statement like the Mihail—Vazirani conjecture holds: we will prove that the expansion of the edge-graphs of half-integral zonotopes is uniformly bounded away from $0$. This is obtained by showing as an intermediate result that half-integral zonotopes are always graphical. This talk is based on joint work with Jean Cardinal.
Christophe Roux
Jan-Dirk Schmöcker
Kyoto and many other cities face a serious “Overtourism” problem. To find solutions, good models of estimating tourist flows within a city are helpful. Solutions to distribute traffic flows are then, however, still difficult to achieve as many attraction points are considered “must visits”. The talk will combine the conference theme by firstly describing Deep Learning approaches and models based on entropy maximisation ideas to estimate flows. In the latter part the talk will then discuss some (discrete) dynamic pricing strategies to optimize the usage of an existing bus fleet serving key attractions in a city.
Martin Skutella
Neural networks with ReLU activation play a key role in modern machine learning. Understanding the functions represented by ReLU networks is a major topic in current research as this enables a better interpretability of learning processes. Injectivity plays a crucial role whenever invertibility of a neural network is necessary, such as, e.g., for inverse problems or generative models. The exact computational complexity of deciding injectivity was recently posed as an open problem (Puthawala et al. [JMLR~2022]). We answer this question by proving coNP-completeness. On the positive side, we show that the problem for a single ReLU layer is still tractable for small input dimension; more precisely, we present a parameterized algorithm which yields fixed-parameter tractability with respect to the input dimension. In addition, we study the network verification problem which is of great importance since neural networks are increasingly used in safety-critical systems. We prove that network verification is coNP-hard for a general class of input domains. Our result thus highlights that the hardness of network verification is intrinsic to the ReLU networks themselves, rather than specific input domains. In this context, we also characterize surjectivity for ReLU networks with one-dimensional output which turns out to be the complement of a basic network verification task. We reveal interesting connections to computational convexity by formulating the surjectivity problem as a zonotope containment problem. This is joint work with Vincent Froese and Moritz Grillo.
Christoph Spiegel
We discuss recent progress on using neural networks as approximators for mixed discrete-continuous problems in extremal combinatorics such as the Hadwiger-Nelson problem or problems involving Graph Limits.
Bartolomeo Stellato
We present computational tools to analyze and design first-order methods in parametric convex optimization. First-order methods are popular for their low per-iteration cost and warm-starting capabilities. However, precisely quantifying the number of iterations needed to compute a high-quality solution remains a key challenge, especially in safety-critical applications where real-time execution is crucial. In the first part of the talk, we introduce a numerical framework for verifying the worst-case performance of first-order methods in parametric quadratic optimization. We formulate the verification problem as a mathematical optimization problem that maximizes the fixed-point residual at the last iteration, subject to constraints representing the algorithm used. Our framework can encode many proximal algorithms as variations of two primitive steps: affine steps and element-wise maximum steps. We show that the verification problem is NP-hard, and we construct both mixed-integer linear programming formulations and convex semidefinite programming relaxations. Numerical examples show a significant reduction in pessimism compared to standard worst-case convergence analyses and performance estimation techniques. In the second part of the talk, we present a data-driven approach to analyze the performance of first-order methods using statistical learning theory. We build generalization guarantees for classical optimizers, using sample convergence bounds, and for learned optimizers, using the Probably Approximately Correct (PAC)-Bayes framework. We then use this framework to learn accelerated first-order methods by minimizing the PAC-Bayes bound directly over the key parameters of the algorithms (e.g., gradient steps, and warm-starts). Several numerical experiments show that our approach provides strong generalization guarantees for both classical and learned optimizers with statistical bounds that are very close to the true out of sample performance.
Tamon Stephen
The Fredman-Khachiyan algorithm generates the transversal of a hypergraph in incremental quasi-polynomial time. It is a recursive algorithm which focuses on the most frequent vertex in terms of the number of edges in either the hypergraph or its transversal. Hypergraphs where this maximum frequency is low are therefore of interest. This gives a group of related optimization questions for hypergraph-transversal pairs. Here we present some preliminary extremal results. Besides using classic combinatorial constructions, we adapt Wagner’s deep reinforcement learning scheme from graphs to hypergraphs to help find some unexpected examples.This is joint work with Parsa Salimi.
Masashi Sugiyama
Standard supervised machine learning requires correctly labeled data. However, correcting such correct labels is often expensive or even impossible in practical applications. In this talk, we discuss a particular weakly supervised learning scenario called complementary classification, which tries to train a classifier from wrongly labeled data.
Kim-Chuan Toh
Doubly nonnegative (DNN) programming problems are known to be challenging to solve because of their huge number of $\Omega(n^2)$ constraints and $\Omega(n^2)$ variables. In this work, we introduce RiNNAL, a method for solving DNN relaxations of large-scale mixed-binary quadratic programs by leveraging their solutions’ possible low-rank property. RiNNAL is a globally convergent Riemannian based augmented Lagrangian method (ALM) that penalizes the nonnegative and complementarity constraints while preserving all other constraints as an algebraic variety. After applying the low-rank decomposition to the ALM subproblem, its feasible region becomes an algebraic variety with favorable geometric properties. Our low-rank decomposition model is different from the standard Burer-Monteiro (BM) decomposition model in that we make the crucial step to equivalently reformulate most of the quadratic constraints after the BM decomposition into fewer and more manageable affine constraints. This modification is also important in helping us to alleviate the violation of Slater’s condition for the primal DNN problem. Moreover, we make the crucial step to show that the metric projection onto the algebraic variety, although non-convex, can be transformed into a solvable convex optimization problem under certain regularity conditions. Numerous numerical experiments are conducted to validate the efficiency of the proposed RiNNAL method. Joint work with Di Hou and Tianyun Tang
Berkant Turan
We explore and formalize backdoor-based watermarks and adversarial defenses, framing them as interactive protocols between two parties. Our findings reveal that for almost any learning task, at least one of the two schemes is feasible. We also uncover a third, essential scheme — transferable attacks — that allows attackers to craft queries that are indistinguishable from the true data distribution, but successfully attack any efficient defender. Using a cryptographic construction with homomorphic encryption, we demonstrate the necessity of transferable attacks and establish their connection to fundamental cryptographic principles.
Andrea Walther
Frank-Wolfe methods are very well studied for the smooth convex case. Recently, we extended this analysis for the convex, piecewise smooth case. We will discuss convergence rates for different situations. Furthermore, we present numerical results that were obtained with the Julia-based implementation AbsSmoothFrankWolfe.jl to verify the theoretical results.
Yao Xie
We consider the variable selection problem for two-sample tests, aiming to select the most informative variables to distinguish samples from two groups. To solve this problem, we propose a framework based on the kernel maximum mean discrepancy (MMD). Our approach seeks a group of variables with a pre-specified size that maximizes the variance-regularized MMD statistics. This formulation also corresponds to the minimization of asymptotic type-II error while controlling type-I error, as studied in the literature. We present mixed-integer programming formulations and develop exact and approximation algorithms with performance guarantees for different choices of kernel functions. Furthermore, we provide a statistical testing power analysis of our proposed framework. Experiment results on synthetic and real datasets demonstrate the superior performance of our approach. This is a joint work with Jie Wang, and Santanu Day at Georgia Tech.
Haoxiang Yang
Multistage stochastic mixed-integer programs (MS-MIP) with general mixed-integer state variables are considered computationally challenging to solve. Lagrangian cuts on lifted state spaces can adaptively approximate the nonconvex value functions. However, generating Lagrangian cuts is time consuming as it requires solving Lagrangian relaxation problems iteratively. We develop a learning-to-cut scheme within the cutting-plane algorithm to quickly obtain the dual multiplier close to optimal ones. Comparative analyses reveal that our learning-to-cut algorithm outperforms benchmark algorithms, showcasing the potential of machine learning to tackle the complexities of MS-MIP effectively.
Yuriy Zinchenko
The analysis of why a specific MIP instance is infeasible formally can be reduced to computing an Irreducible Infeasible Subset (IIS) of the constraints. Unlike the case of LP, for MIP there is no useful duality that can be employed to facilitate such computations. The process of determining an IIS for MIP is typically handled with brute force, e.g., by use of deletion filters and alike, thus rendering IIS determination for a MIP into a much harder computational task. We will discuss one approach to optimizing this process and what components of this approach could make it into the newest version of Gurobi.
Previous Editions
[ DO x ML 2018 | DO x ML 2019 | DO x ML 2020 | DO x ML 2021 | DO x ML 2023 | DO x ML 2024 ]